Business Growth with Predictive CLV Models
Unlock customer insights with predictive CLV models—strategies to drive growth
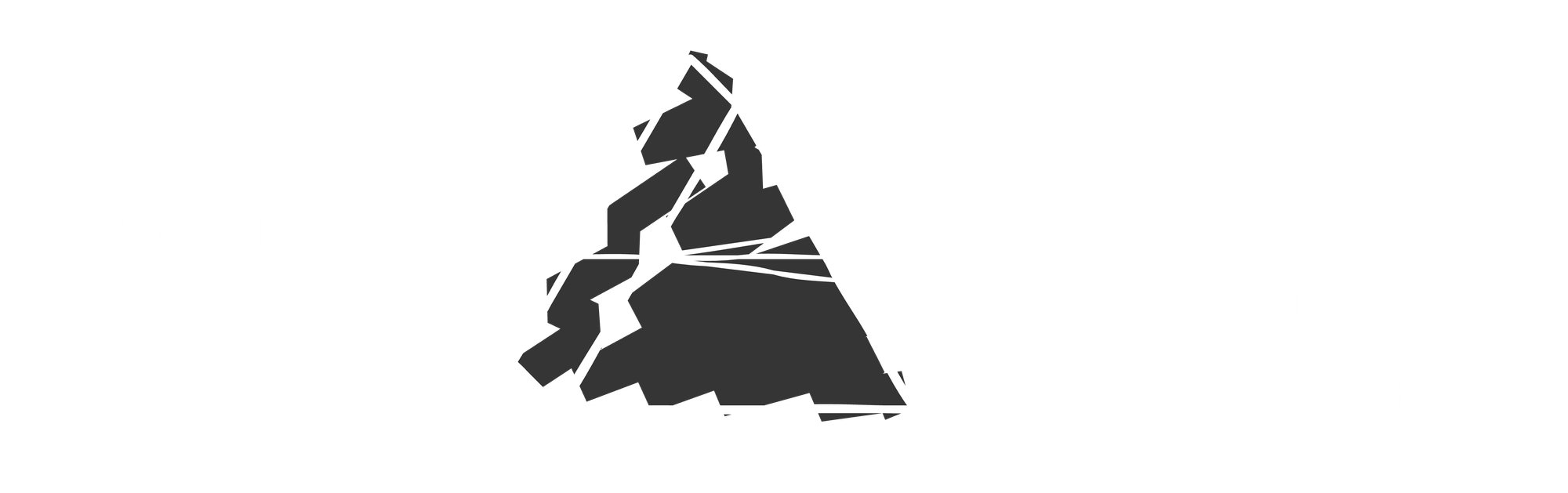
Customer relationships are more valuable than ever. For companies aiming to grow sustainably, understanding each customer’s lifetime value (CLV) is crucial. CLV is a metric that estimates the total revenue a business can expect from a customer over the entire duration of their relationship. Far from being a one-time measurement, CLV provides an evolving, strategic lens that helps companies assess which customers contribute most to long-term success. By focusing on maximizing CLV, companies can shift their resources toward nurturing high-value relationships, ultimately building a customer base that drives consistent growth.
Predictive CLV models bring this concept into action, enabling businesses not only to understand current customer value but to forecast future value accurately. By analyzing historical data, purchase patterns, and customer behaviors, these models use advanced analytics to project the future revenue a customer might bring. This predictive power allows companies to make more informed decisions about where to invest marketing dollars, how to tailor their retention strategies, and even which customer segments to prioritize. Ultimately, predictive CLV models serve as a foundational tool for optimizing marketing spend, strengthening customer loyalty, and enhancing overall profitability.
Predictive CLV models have the potential to revolutionize customer management and strategic planning. By transforming data into actionable insights, these models enable businesses to move beyond a transactional approach and focus on building lasting, high-value relationships. In doing so, predictive CLV models offer companies a path to growth that is not only data-driven but also profoundly customer-centric, positioning them to lead in today’s competitive market.
Understanding Predictive CLV Models
Predictive CLV models are advanced analytical tools that estimate the future value of a customer by assessing how much revenue they are likely to generate over their relationship with the company. Unlike traditional CLV calculations, which look backward at historical data, predictive CLV models leverage this data to anticipate future customer behavior. These models rely on sophisticated analytics, incorporating customer purchase history, transaction frequency, and engagement metrics to create a forecast. Predictive CLV allows companies to make proactive decisions, directing resources to customers likely to yield high returns and enhancing the overall value of their customer base.
Types of Predictive Models:
Various predictive approaches can be used to model CLV, each with strengths for analyzing customer behavior:
- Neural Networks: Deep learning models, such as neural networks, are highly effective for capturing complex, nonlinear relationships within large datasets. These models identify intricate patterns in customer behavior, such as the influence of multiple interactions on long-term value.
- Machine Learning Algorithms: Techniques like Random Forests and Gradient Boosting use decision trees to analyze diverse customer segments and optimize predictions for different customer types. Ensemble models, for example, combine several algorithms to improve accuracy, making them particularly useful for CLV predictions that require segmentation .
Key Metrics for Prediction
Predictive CLV models commonly integrate several customer-centric metrics, each contributing unique insights into long-term value:
- Net Promoter Score (NPS): NPS gauges customer loyalty by asking, “On a scale of 0–10, how likely are you to recommend our company to a friend or colleague?” Higher NPS scores correlate with higher CLV, as loyal customers tend to make more repeat purchases and offer referrals. NPS can be incorporated into the predictive model as follows:
[]
Where:
- Promoters: Customers scoring 9–10.
- Detractors: Customers scoring 0–6.
- Average Transaction Value (ATV): ATV indicates the average amount a customer spends per transaction. Increases in ATV directly contribute to CLV, as higher transaction amounts yield greater revenue. ATV can be calculated as:
[]
- Customer Effort Score (CES): CES measures the ease with which customers interact with a company’s products or services. Lower CES scores (indicating less effort required by customers) are associated with higher CLV, as these customers are more likely to remain loyal and engage in future transactions. CES can be calculated as:
[]
Importance of Integrating Metrics
Combining NPS, ATV, and CES in predictive CLV models provides a multidimensional view of customer value. Each metric offers insights into different facets of customer behavior—NPS reflects loyalty, ATV captures spending potential, and CES assesses ease of interaction. By integrating these metrics, companies can enhance their predictive models, creating a comprehensive view of customer segments and enabling more effective targeting and retention strategies.
A simple approach to combine these metrics in a predictive CLV formula could look like:
[]
Each weight can be determined based on the significance of each metric for the specific business context, allowing for model customization.
This approach allows predictive CLV models to harness the power of multiple customer-centric metrics, resulting in a nuanced view of customer value that enhances segmentation, retention, and strategic decision-making.
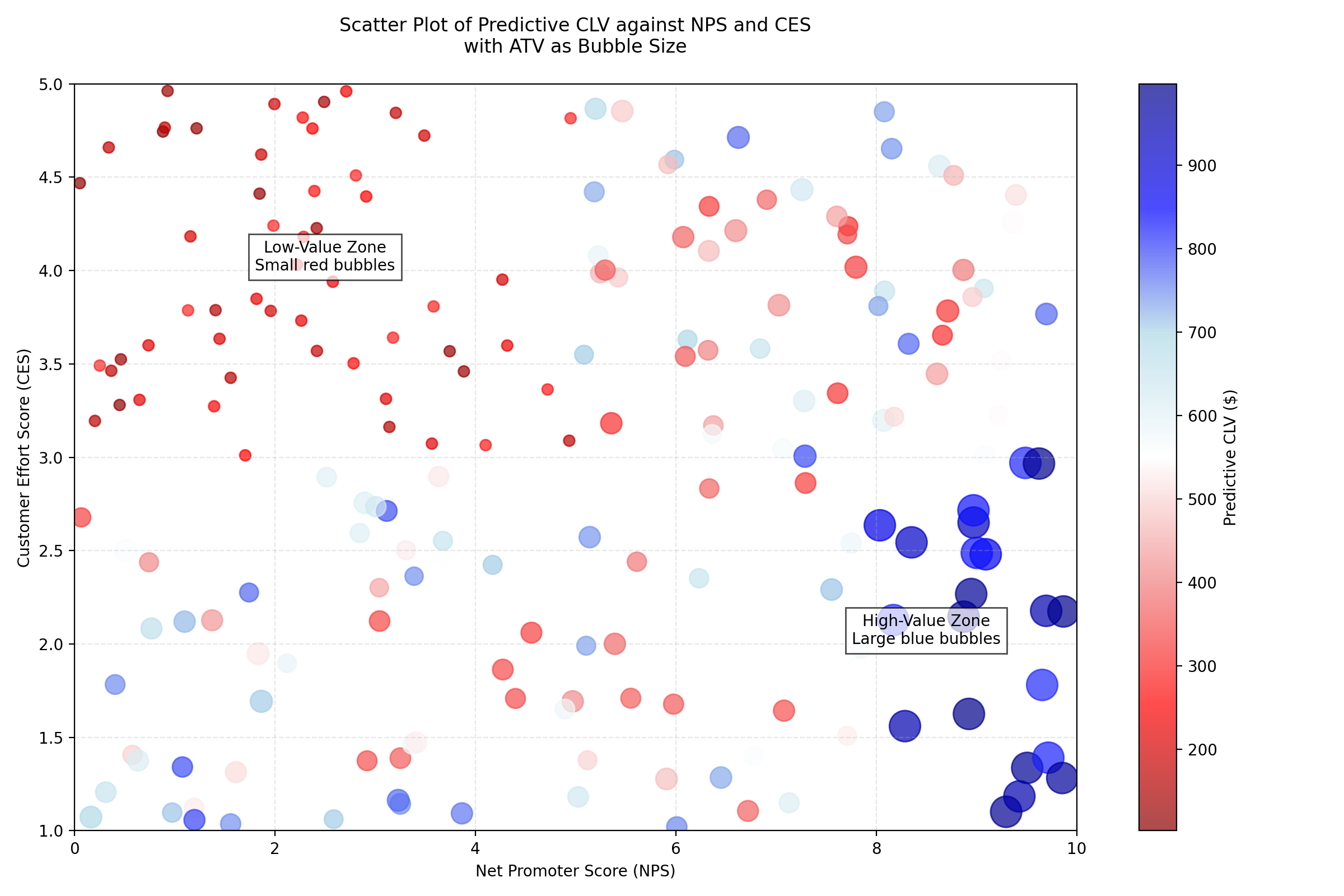
This scatter plot represents an analysis of Predictive Customer Lifetime Value (CLV) conducted for a consulting customer, a Data-as-a-Service (DaaS) platform based in Colombia with a customer base of 11,000. The platform offers a portfolio of seven different apps and services.
The analysis segmented customers based on their Net Promoter Score (NPS) and Customer Effort Score (CES), with bubble sizes indicating Average Transaction Value (ATV) and color intensity reflecting Predictive CLV.
- High-Value Zone (Right): The larger, blue bubbles in the top right corner represent high-value customers with high loyalty (NPS 8-10) and low effort (CES 1-3), ideal targets for retention and loyalty programs.
- Low-Value Zone (Left): The smaller, red bubbles indicate customers with low loyalty and high effort, potentially indicating areas for service improvement.
Thanks to insights from this predictive CLV analysis: Annual Recurring Revenue (ARR) increased by 18%; Cross-sell and upsell efforts saw a 3.7x increase, effectively boosting customer value and engagement.
The Strategic Importance of CLV: a metric of sustained hyper-growth prediction
Customer Lifetime Value (CLV) is more than just a financial metric; it’s a strategic asset that highlights the revenue potential of long-term customer relationships. High-CLV customers often drive a substantial portion of a company’s revenue, making them critical to sustained growth. These customers tend to make repeat purchases, contribute larger transaction values, and are often more responsive to loyalty programs. By identifying and nurturing high-CLV customers, companies can ensure a steady revenue stream and reinforce the value of customer-centric strategies. This focus on high-CLV customers shifts a company’s attention from one-off transactions to long-term value, helping to build a stable foundation for growth.
CLV in Strategic Decision-Making:
CLV provides a quantitative basis for key decisions, from budgeting to marketing and beyond. By understanding the lifetime value of different customer segments, businesses can allocate resources more efficiently, optimize spending, and increase profitability.
• Customer Acquisition and Retention:
CLV enables companies to optimize their marketing budgets by distinguishing between high-value and low-value customers. With predictive CLV models, businesses can target acquisition efforts towards potential high-CLV customers, maximizing return on investment in customer acquisition. Additionally, CLV insights guide retention strategies, helping companies to invest in initiatives like loyalty programs or personalized offers that keep high-value customers engaged and satisfied.
• Segmentation and Prioritization:
Predictive CLV insights allow companies to segment their customer base based on expected future value. This enables businesses to prioritize high-CLV segments, ensuring that the most valuable customers receive more attention and resources. By aligning marketing strategies with these insights, companies can create tailored campaigns that resonate with different segments, fostering loyalty and maximizing each segment’s potential. Prioritization based on CLV can significantly improve resource allocation, making marketing efforts more efficient and impactful.
Alignment with Market Orientation Goals:
A CLV-focused strategy inherently aligns with market orientation goals by centering business efforts around customer satisfaction and loyalty. By focusing on customers who bring long-term value, companies can better anticipate customer needs and adapt their offerings to enhance satisfaction. This approach benefits overall business health by fostering a customer-first mentality that not only boosts revenue but also strengthens brand loyalty. In turn, this alignment with market orientation helps businesses achieve sustained growth, as a satisfied customer base provides consistent, reliable revenue while reducing the need for constant new customer acquisition.
CLV is a strategic tool that empowers businesses to focus on sustainable, customer-centered growth. By prioritizing high-value customers, aligning resources accordingly, and building strategies that reinforce customer satisfaction, companies can enhance profitability while strengthening their brand in the marketplace.
Conclusion
Predictive CLV models offer powerful tools for enabling customer-centric growth. By accurately forecasting customer value, these models help businesses prioritize high-value customers, optimize marketing spend, and enhance retention efforts. Companies can build lasting relationships and drive consistent revenue growth through targeted, data-driven strategies.
As customer expectations and market dynamics continue to shift, predictive CLV models will need to be continuously optimized. Regular updates and refinements will ensure that these models stay relevant, offering actionable insights that align with evolving customer behaviors.
Businesses should consider investing in predictive CLV models to harness the full potential of customer data. With the ability to make informed, customer-focused decisions, companies can strengthen their market position, drive strategic growth, and achieve sustainable success in today’s competitive landscape.